The Role Of AI In Creating an Inclusive Credit Underwriting Policy It is important to partner with a provider that runs rigorous testing on every loan and application to be fair to all the applicants with direct oversight from regulators and other expert stakeholders
By Nitin Sharma •
Opinions expressed by Entrepreneur contributors are their own.
You're reading Entrepreneur India, an international franchise of Entrepreneur Media.
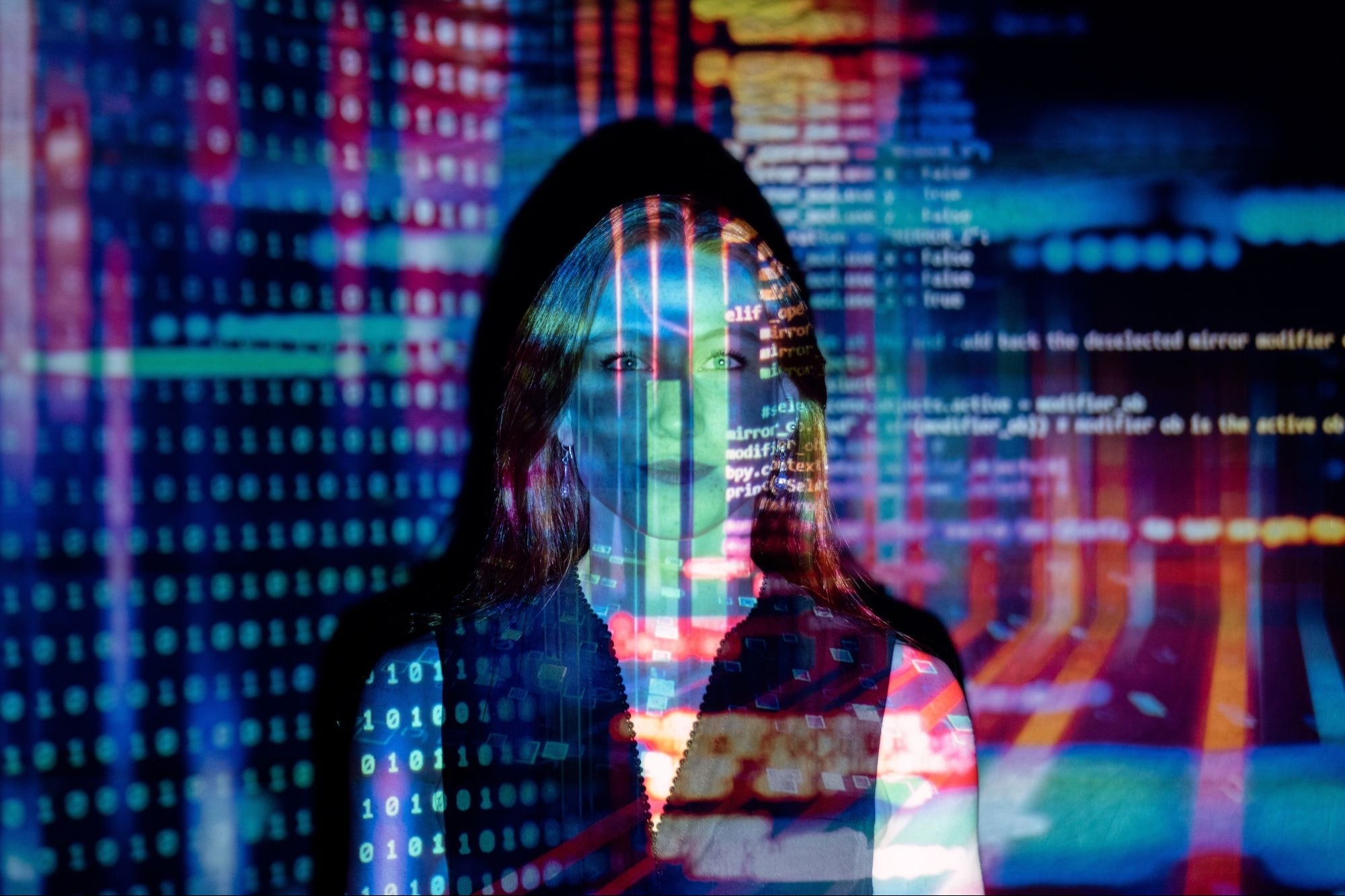
Are the current credit underwriting policies not inclusive?
They may not be, as these policies are drafted by human beings, like you and me, who are inherently biased and therefore prone to making rules that may discriminate against certain individuals or communities without the intention to do so.
To address this issue effectively, the federal law in the US makes it illegal for a lender to deny credit or offer different terms based on protected traits like race, color, or religion.
But do we have the same rules in India?
As of today, we, unfortunately, don't have any such specific regulations in place.
Does it mean there is no way to keep a check on credit and lending discrimination in India?
Considering we're right now living in the golden age of financial technology, we can now explore other ways to control the discrimination born out of manually checking creditworthiness.
If banks and other financial institutions want to reach the 'credit invisible' population and bring them into the formal financial system, they need to adapt to a more advanced technological infrastructure than what they have now.
Which is, of course, easier said than done.
But this is where technology comes to our rescue!
The next step toward giving more people access to money is to use credit ratings that aren't based on credit reports but on data from smartphones.
Biases that influence credit underwriting policy
Traditional lending institutions rely on the credit bureaus' use of covert algorithms to figure out credit scores, which is their main source of income. Credit scoring in India is severely hampered by massive issues resulting from data delays.
They also tend to show biases from the past and are usually not very representative because the data they use is biased.
Women, for example, have a harder time getting credit than men do, even though their credit histories are much better.
Similarly, if we look at recent college graduates and young people with short credit histories, they have a hard time building up a credit history.
To address this, credit bureaus are rapidly going digital to produce real-time, data-driven scoring. This could make it easier to figure out a borrower's risk when they are already part of the financial system. But it would still make it hard for people without credit histories, which is about 190 million Indians, to get money.
Lending biases are already prevalent due to the difficulty in vetting potential borrowers. Financiers often use a weighted average of loan interest rates when they don't have any good risk models.
What's the result? A lower-than-appropriate interest rate for average borrowers and a higher-than-appropriate rate for prime borrowers.
As a result of these flawed lending systems, lenders often end up giving credit to the wrong people, and a significant population never gets the chance to build up the data needed to secure a loan in the future.
How do AI and ML help in creating an inclusive underwriting policy?
To get rid of the biases that have been seen in lending so far, lenders today are putting a lot of emphasis on AI and alternative data. New studies from LSU and Harvard demonstrate that if lenders use AI and alternative data like education and employment history, many more people could qualify.
The concept allows for continuous monitoring of a borrower's fiscal status and spending habits. How? Modern digital lenders build customer profiles by using information from nontraditional sources like customers' contact lists, text messages, web browsing histories, and use of mobile apps to get a fuller picture of how borrowers spend their money.
With the help of AI and ML, these predictive risk assessment models also help take into account a variety of factors and get rid of biases.
Using AI and ML, loan applications can be evaluated both quantitatively and qualitatively. Qualitative evaluations look at things that the borrower has no control over but that may affect how likely the application is to be approved.
When you look closely at GST information, you can learn a lot about the borrower's main business, how the company has grown and changed in the past, how reliable the borrower is, and more.
Until recently, lenders had to check a lot of documents to make sure an applicant was who they said they were, figure out if they had good credit, and then give them a secured loan. However, AI works to address this problem for those who otherwise would not be able to meet these requirements.
Using tiered KYC [Know Your Customer] verification processes, AI is helping to solve identity problems in two ways: by recognizing faces and putting together data from digital activities. This means that a person's social network, call history, and even mobile phone top-ups can all be used as additional information about them.
Financial service providers are using artificial intelligence to help make financial inclusion a reality in emerging countries where mobile phone use and penetration of ICTs are both on the rise.
Data mining can fix the problems with traditional credit scoring by using easy-to-check criteria like monthly utility bills, emails, use of social media, contact lists, GPS data from cell phones, and psychological factors measured by psychometric testing.
In conclusion
To sum up, we can say that AI and ML can change a credit system that was biased and excluded a large portion of people. Critics, at the same time, say that AI and ML can also be biased, especially when there isn't much data to train them on.
That is why it is important to partner with a provider that runs rigorous testing on every loan and application to be fair to all the applicants with direct oversight from regulators and other expert stakeholders. By using the opportunities offered by artificial intelligence and machine learning, credit unions can help make the financial system fairer and easier for more people to use.